When evaluating and measuring your Facebook campaigns that utilize Campaign Budget Optimization (CBO) and Lowest Cost Bidding Strategy, performance should be evaluated in terms of the sum of the whole, not the individual ad sets and ads.
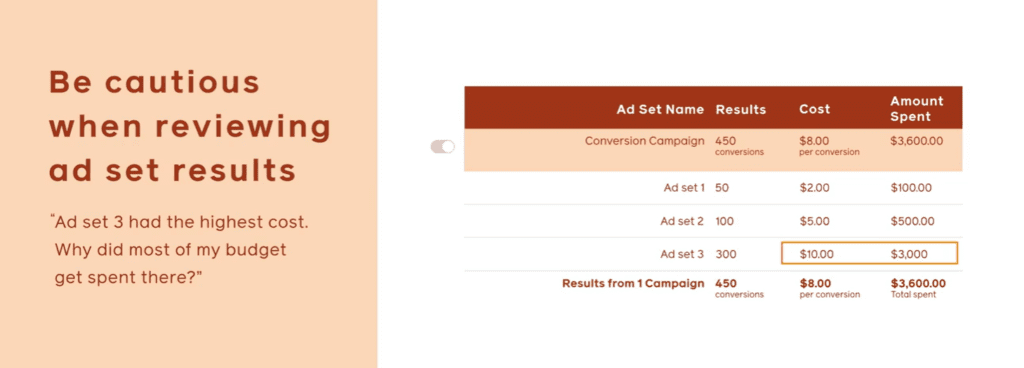
As Meta explains, when individuals review a report like the one above, a common misconception is that Meta’s machine learning moves more budget to underperforming ad sets – a phenomenon dubbed “The Breakdown Effect.”
In reality, this is not the case: it only appears so because marketers aren’t exposed to the real-time costs of results in the auction (the machine learning system that takes place to determine which ad is shown to a person). For example, it may look odd that an ad set with a higher cost per conversion was given more budget (as above), but this was likely because the auction system determined that the cost per conversion of the other ad sets would increase if given more money.
Campaign Budget Optimization with Lowest Cost Bid Strategy enabled allows the system to dynamically shift budget to ad sets and ads that will result in the highest number of results for your campaign. This is the opposite of assigning specific ad set budgets, or giving specific budgets to specific audiences, in a single campaign.
“The Breakdown Effect” gets to the heart of how Meta’s auction system works, and can be a common point of confusion. It’s important for marketers to understand this concept so they can discern why reports may look a certain way, and so they aren’t tempted to turn off CBO by assigning specific budgets to specific entities in the same campaign to drive more budget to entities with lower costs. This will deliver fewer results and an overall higher cost per result for your campaign.
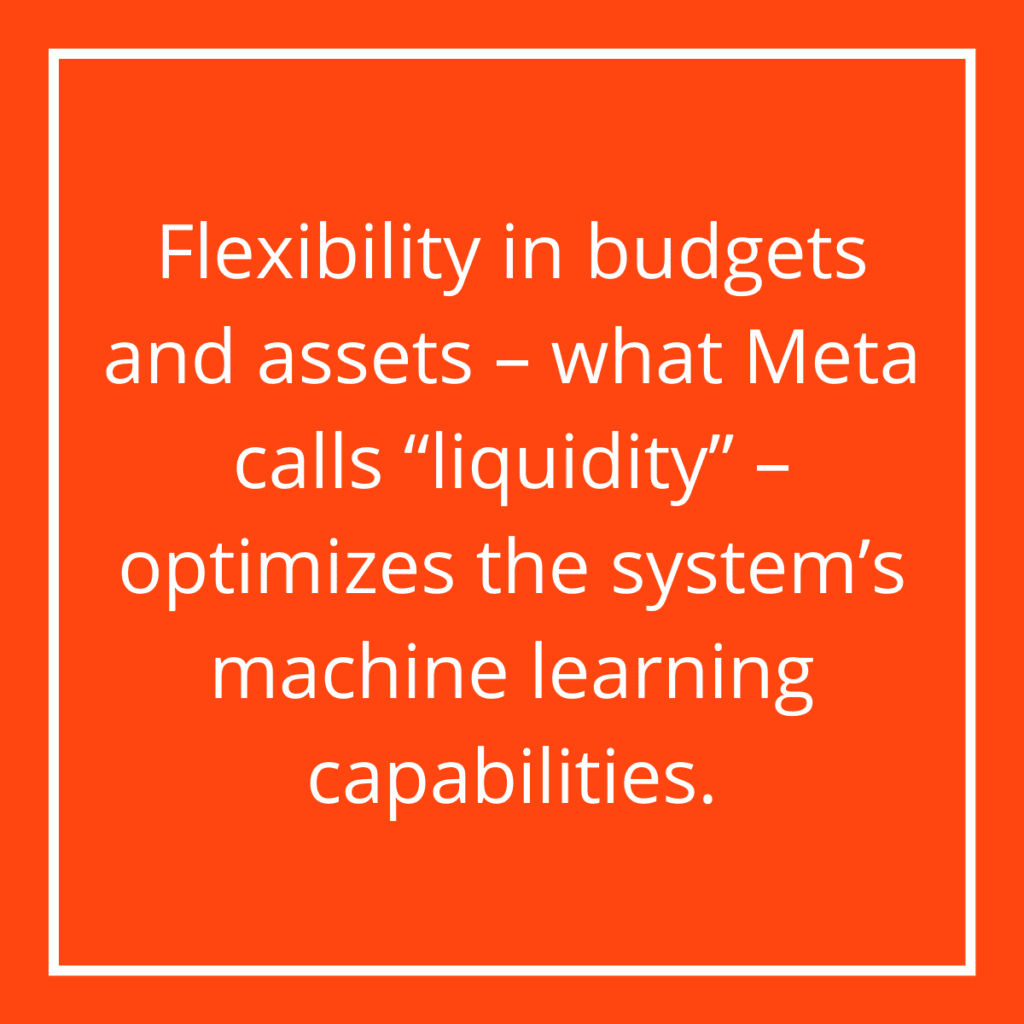
For brands with nimble budgets, it can be tempting to make every dollar count by designating dollars to specific ad groups based on previous performance costs. But doing so based on an evaluation of campaigns using CBO will deliver the opposite effect because individual ad sets & ads should not be viewed in isolation as performance costs of individual entities in a CBO campaign are not the best indicator of success. And, also because this limits the system’s ability to learn and move budget in a fluid manner based on real-time happenings in the auction. Flexibility in budgets and assets – what Meta calls “liquidity” – optimizes the system’s machine learning capabilities.
Remember, the more flexibility, the better as the cost per result of a specific entity in CBO campaign is not a good indicator of success. While reviewing overall campaign performance of a CBO campaign is ideal, the amount of budget assigned to different entities is a better indicator of success when trying to evaluate different ads and ad sets.
If you need assistance in how to structure and optimize budgets to the platform’s machine learning capabilities with CBO, please contact us.